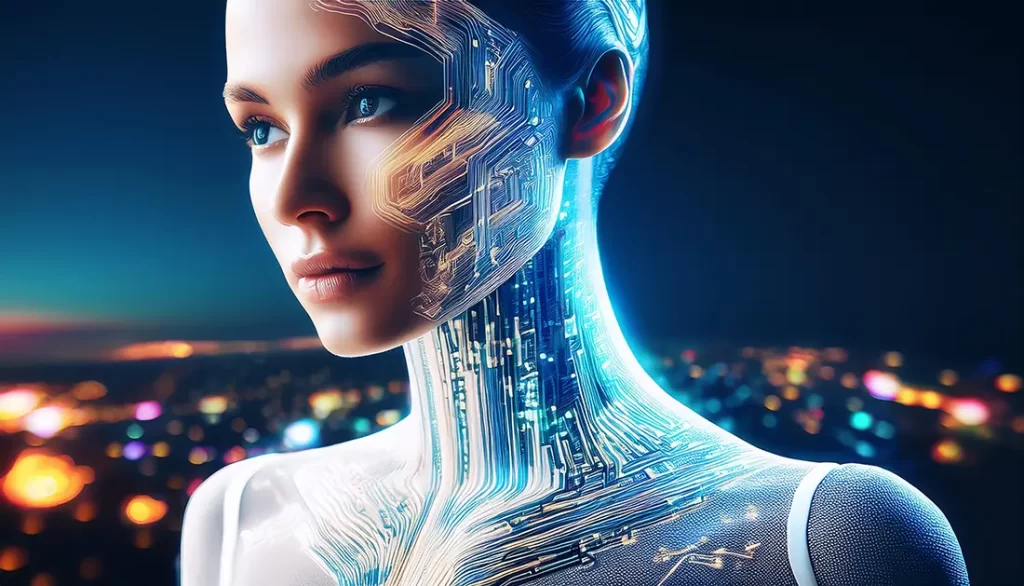
1. Ethical Foundations for AI Development
- Bias Mitigation: Rigorous testing and diverse datasets to prevent AI from reinforcing or introducing biases.
- Transparency and Accountability: Clear documentation of AI processes and accountability for outcomes to foster public trust.
- AI for Social Good: A proactive focus on applications that promote human well-being, sustainability, and equality, avoiding uses that could harm society.
2. Global Cooperation and Standardization
- International Standards: Universal standards for ethical AI practices, data sharing, and cybersecurity to build trust across borders.
- Cross-Border Collaboration: Shared AI initiatives targeting global issues like climate change, health, and inequality to enable collective progress.
3. Environmental Sustainability in AI
- Energy-Efficient AI Models: Prioritize the development of models that require less computational power, and explore renewable energy solutions.
- Offsetting AI’s Carbon Footprint: Encourage AI organizations to invest in sustainable practices, such as carbon offsets, to mitigate the ecological impact.
4. Digital Inclusion and AI Accessibility
- Bridging the Digital Divide: Investment in AI infrastructure and literacy programs in under-resourced areas to democratize access.
- AI Education and Reskilling: Initiatives to educate all demographics on AI, ensuring society-wide engagement and understanding.
5. AI’s Role in Society and Governance
- Public Involvement in AI Policy: Policymakers should engage communities most impacted by AI to ensure ethical considerations are met.
- AI-Enhanced Democracy: AI can improve democratic processes, but transparency is vital to prevent manipulation.
6. Focus on AI for Social Impact
- Global Challenges: AI should prioritize addressing health, environmental sustainability, and equality through applications like those already seen with initiatives combating deforestation or optimizing renewable energy.
7. Human-AI Collaboration and Workforce Transformation
- Hybrid Roles: AI will introduce roles that combine human creativity with machine precision. Training and reskilling are key to preparing the workforce for these changes.
Section 1
1. Ethical Foundations for AI Development
Bias Mitigation
- Diverse and Inclusive Datasets: Ensuring datasets are representative across demographics (race, gender, age, socioeconomic status) is critical to avoid skewed outputs.
- Continuous Auditing: AI models should be routinely audited for fairness across use cases. This includes identifying potential biases that may not have been apparent during initial testing.
- Feedback Mechanisms: Creating feedback loops from users can help detect and address unforeseen biases, especially when AI is deployed in dynamic environments.
Transparency and Accountability
- Explainable AI (XAI): AI systems should be designed to be interpretable. For example, an AI’s decision path could be presented as a series of logical steps, allowing users to understand and question outputs.
- Clear Responsibility Chains: Establishing accountability by linking AI outcomes to specific team members or departments responsible for oversight, preventing diffusion of responsibility.
- Incident Reporting: When AI systems cause harm or malfunction, a transparent incident report system should be in place to learn from these occurrences and prevent future risks.
AI for Social Good
- Impact Assessments: AI projects should undergo an assessment to evaluate their potential impact on social good versus harm. This process can help organizations decide whether to pursue certain AI applications.
- Priority for Sustainability and Equality: AI-driven projects focused on environmental sustainability, health, and equality should receive priority in both funding and development. This supports a forward-looking approach where AI contributes positively to society.
- Limiting Harmful Applications: Applications that could infringe on privacy, manipulate public opinion without transparency, or disproportionately affect vulnerable populations should be restricted or closely monitored.
Expansion Ideas:
- Ethical Oversight Boards: Each AI project could benefit from an independent, diverse board providing oversight to ensure ethical guidelines are being followed. These boards would ideally include ethicists, community representatives, and technical experts.
- Ethics Training for AI Developers: Offering ethics training specifically tailored to AI development could enhance the awareness of ethical considerations among developers, reducing inadvertent ethical oversights.
Section 2
2. Global Cooperation and Standardization
International Standards
- Unified Ethical Guidelines: Establishing a baseline for ethical practices that all nations adhere to, covering areas like privacy, data protection, and transparency. This ensures that AI developments align with a shared set of values, minimizing ethical discrepancies across borders.
- Interoperability Standards: Standards for AI models and data that allow seamless integration and communication across systems and countries. This can reduce inefficiencies and ensure that AI solutions developed in one region are compatible globally.
- Regulatory Harmonization: Governments and regulatory bodies should aim for compatible regulatory frameworks, making it easier for AI companies to operate internationally while upholding ethical standards.
Cross-Border AI Initiatives
- Joint AI Research for Global Challenges: Collaborative AI research initiatives could target critical global issues, such as climate change, public health, poverty, and education. Shared access to datasets and resources can allow even nations with fewer AI capabilities to benefit and contribute.
- Global AI Resource Sharing: Establishing programs where more developed AI nations share AI resources, like open datasets, computing power, and expertise, with developing countries. This could promote equitable access and reduce the risk of AI being concentrated in a few regions.
- Disaster Response and Resilience: Creating an international AI framework for responding to global crises (natural disasters, pandemics) where AI models are pooled and optimized for response efforts. This could include shared surveillance, predictive modeling, and resource allocation tools.
Cybersecurity and Data Privacy Protocols
- Unified Data Privacy Policies: Establishing international agreements on data privacy to protect individuals across borders. This includes clear guidelines on data usage, anonymization standards, and consent practices.
- Cybersecurity Collaboration: Countries could share AI-driven cybersecurity practices and threat intelligence to counteract cyber threats more effectively. This collaboration would include regular reporting, knowledge sharing, and rapid response teams for AI-related security threats.
Promoting Equitable Access to AI
- Funding for AI in Underserved Regions: Providing grants and support to develop AI infrastructures in underserved areas can create a more balanced global AI landscape, reducing inequalities.
- Educational Partnerships: International AI education programs to equip all regions with the knowledge and skills necessary to develop and govern AI responsibly.
Expansion Ideas
- Global Ethical AI Body: Creating an international governing body to monitor AI developments, enforce ethical standards, and mediate cross-border disputes regarding AI practices.
- Cross-Cultural AI Development: Encourage multi-cultural research teams, which could help develop AI systems that respect and reflect the values of different regions, avoiding one-size-fits-all approaches.
Section 3
3. Environmental Sustainability in AI
Energy-Efficient AI Models
- Model Optimization: Techniques like pruning, quantization, and knowledge distillation can reduce model complexity and computational demands without significantly impacting performance.
- Algorithm Efficiency: Focusing on creating algorithms that achieve desired outcomes with minimal computation can save energy. This includes exploring more efficient architectures and training methods.
- Federated Learning: By processing data locally on devices rather than in data centers, federated learning can reduce the need for extensive cloud computing, thereby cutting down on energy consumption.
Use of Renewable Energy
- Transitioning to Green Data Centers: Encouraging AI companies and research institutions to rely on renewable energy sources for powering data centers can significantly reduce their carbon footprint.
- Energy Offset Initiatives: AI organizations could invest in renewable energy projects or carbon offset programs to balance out their environmental impact. This could be structured as part of their corporate social responsibility.
Sustainable AI Hardware
- Recyclable and Energy-Efficient Hardware: Encouraging the use of recyclable materials in AI hardware manufacturing can make AI’s physical components more sustainable. Additionally, energy-efficient hardware, such as advanced GPUs designed for low power consumption, can reduce overall energy requirements.
- Hardware Longevity: Designing systems with modularity in mind can allow hardware upgrades instead of complete replacements, reducing electronic waste and the energy required to manufacture new hardware.
Offsetting AI’s Carbon Footprint
- Carbon-Neutral Goals: Organizations developing AI could aim for carbon neutrality by setting long-term goals for carbon offsets. This includes committing to practices like reforestation or investing in sustainable agriculture projects.
- Environmental Reporting: AI companies could regularly publish environmental reports detailing their energy use, carbon footprint, and sustainability efforts, promoting transparency and accountability in their environmental impact.
Expansion Ideas:
- Sustainable AI Certifications: Creating certifications for “green AI” could encourage companies to pursue environmentally friendly practices, helping consumers and stakeholders make informed choices.
- AI in Environmental Conservation: Exploring AI applications that directly benefit environmental causes, such as monitoring ecosystems, could help make AI itself a tool for sustainability.
Section 4
4. Digital Inclusion and AI Accessibility
Bridging the Digital Divide
- AI Infrastructure in Underserved Areas: Encourage investment in AI infrastructure—such as internet connectivity, data centers, and accessible devices—in regions with limited resources. This could involve partnerships between governments and tech companies.
- Subsidized Access to AI Tools: Governments and international organizations could provide grants or subsidies to make AI tools and resources affordable in low-income regions, empowering local communities to leverage AI.
AI Education and Training
- Widespread AI Literacy Programs: Establish educational programs at various levels (schools, universities, community centers) that teach fundamental AI concepts, allowing more people to understand and engage with AI.
- Vocational Training for AI Skills: Developing specialized training for in-demand AI skills, including data handling and machine learning, can help workers enter AI-related fields. This would support both workforce transformation and wider inclusion.
- Policy and Decision-Maker Training: Ensure that policymakers and leaders understand AI basics so they can make informed decisions about regulation, application, and societal impacts.
Accessible AI Design
- User-Friendly Interfaces: Design AI applications with user experience in mind, particularly for those with limited technical knowledge, to maximize usability.
- Multilingual and Culturally Sensitive AI: Building AI systems that respect cultural nuances and support multiple languages can ensure broader accessibility and inclusivity.
Support for Localized AI Development
- Localized AI Solutions: Encourage AI applications tailored to address local challenges, such as farming assistance in rural areas or education in remote locations.
- Community-Driven AI Projects: Foster community participation in AI development by involving local voices in AI research and solution design, creating tech that reflects and meets regional needs.
Expansion Ideas:
- Global Digital Equity Fund: Establish an international fund specifically dedicated to closing the digital divide, supporting AI initiatives in underserved areas.
- Localized Knowledge-Sharing Networks: Create networks where individuals and organizations can share AI knowledge and resources regionally, creating a collaborative ecosystem for growth.
Section 5
5. AI’s Role in Society and Governance
Public Involvement in AI Policy
- Community Engagement: Government bodies could hold public consultations and workshops to include diverse community perspectives on AI policies. This would ensure AI systems align with societal values and consider potential impacts on various groups.
- Public Education Campaigns: Launch awareness campaigns to help citizens understand the basics of AI, its benefits, and potential risks. This empowers people to participate in the conversation about AI’s role in their lives.
AI-Enhanced Democracy
- Transparent AI in Decision-Making: Governments and organizations could use AI to analyze public opinion and inform policymaking, provided these systems are transparent and the methodology is publicly accessible. This can prevent misuse and increase trust.
- Boosting Civic Engagement: AI can facilitate greater citizen involvement in governance through online platforms, helping people easily access information and provide input on policies and issues relevant to them.
- Preventing Manipulation: Safeguards should be put in place to prevent AI from being used for manipulating public opinion or infringing on personal freedoms. Strict transparency protocols and independent audits can help detect and prevent misuse.
Ethical Oversight of AI
- Independent AI Ethics Committees: Setting up independent, diverse committees that review and guide the ethical implications of AI projects, ensuring they align with societal values and human rights. These committees could have veto power over potentially harmful AI applications.
- Ethics-by-Design Approach: Integrating ethical considerations at every stage of AI development, from design to deployment, ensuring systems are designed with respect for privacy, fairness, and transparency.
- Clear Accountability Structures: Define roles and responsibilities for those involved in AI implementation to ensure there is accountability for its societal impact.
Legal Frameworks and Regulation
- AI Regulation and Legal Standards: Establish regulatory bodies and laws that specifically govern AI applications, ensuring they are safe, ethical, and respect human rights. Regulations should be adaptable to respond to rapid advancements in technology.
- Liability for AI Outcomes: Legal standards should clearly define who is responsible for AI-driven decisions and outcomes, especially in sensitive areas like healthcare, law enforcement, and autonomous vehicles.
- Transparency in AI Audits: Regular audits of AI systems should be required, with findings made public to maintain trust and ensure ongoing compliance with ethical and legal standards.
Expansion Ideas:
- Citizen Panels for AI Projects: Involve randomly selected citizen panels to review and give input on significant AI projects, ensuring community representation in decision-making.
- Ombudsperson for AI Concerns: Establish a public ombudsperson to handle complaints or concerns regarding AI misuse, offering an accessible pathway for the public to voice issues.
Section 6
6. Promoting AI for Social Good
Prioritizing Socially Beneficial AI Applications
- Focus on Global Challenges: Encourage research and funding for AI projects that target critical issues like healthcare, climate change, poverty, and education. AI can significantly impact these areas through predictive modeling, resource allocation, and data analysis.
- Nonprofit Partnerships: AI organizations could partner with nonprofits and NGOs to develop solutions tailored to specific community needs, ensuring technology reaches those who need it most.
- Open-Source AI for Good: Open-source platforms can share AI tools for solving social problems, making resources accessible to researchers, governments, and communities that may lack the budget for proprietary solutions.
Incentivizing Positive Impact Projects
- Grants and Funding for Social Impact: Government and private sector grants could be allocated to projects with measurable social impact, such as environmental monitoring or accessible healthcare technologies.
- Corporate Responsibility Programs: Companies developing AI could establish internal programs to support positive-impact projects, with specific metrics for evaluating social contributions.
Encouraging AI Innovation in Education and Health
- AI-Enhanced Education: Developing AI tools to support personalized education, language learning, and remote access to educational resources can democratize learning and bridge educational gaps.
- AI in Public Health: AI can play a significant role in improving public health by assisting with diagnostics, outbreak prediction, and remote healthcare solutions, especially in under-resourced areas.
AI-Driven Environmental Sustainability Initiatives
- Conservation and Wildlife Monitoring: AI can support environmental conservation through applications like monitoring endangered species, optimizing resource management, and tracking deforestation or ocean health.
- Renewable Energy Optimization: AI tools could optimize renewable energy systems, like wind or solar power, making clean energy more efficient and accessible. AI could also help in waste reduction by optimizing supply chains and consumption patterns.
Public Awareness and Involvement
- Community-Based AI Projects: Engage communities in developing and using AI projects that directly address local issues, allowing residents to have a stake in the solutions.
- Transparency in Social Good Projects: Publicize information on AI projects aimed at social good, ensuring transparency in funding, goals, and outcomes to build trust and inspire further innovation.
Expansion Ideas:
- Social Good AI Award Programs: Establish awards or recognitions for organizations that make outstanding contributions through AI, encouraging a culture of social responsibility.
- Public Database of AI for Social Good: Creating an accessible database of AI projects for social good, categorized by sector, could inspire collaboration and expand awareness of positive applications.
Section 7
7. Human-AI Collaboration and Workforce Transformation
Hybrid Roles in the Workforce
- Blending Human Creativity with Machine Precision: AI’s increasing capabilities will create a new class of hybrid roles where humans and AI work side by side, each bringing unique strengths. These roles will leverage AI’s analytical and computational power alongside human intuition, empathy, and creativity. For example, in fields like design, AI can streamline the technical aspects of concept generation, while humans add insight and artistic vision, creating a synergy that enhances productivity and innovation.
- Co-Pilot Models in Decision-Making: In roles where critical decision-making is essential, AI can act as an analytical co-pilot, providing data-driven insights that support human judgment. This can be particularly valuable in sectors like healthcare, where AI tools can assist in diagnostics and treatment planning, leaving complex ethical decisions to skilled professionals.
Training and Reskilling for AI-Era Jobs
- Ongoing Skill Development: As AI transforms industries, continuous learning programs will be essential for workers to adapt to new tools and workflows. Governments, educational institutions, and private companies can collaborate to create accessible training resources, including online courses and certification programs, that teach skills like data analysis, AI operation, and ethical considerations.
- Reskilling for Emerging Roles: Some traditional roles will evolve or disappear, but new roles will emerge that focus on managing, overseeing, and collaborating with AI systems. Training should focus on preparing workers for positions such as AI ethics officers, data interpreters, and AI maintenance specialists, roles that combine domain expertise with AI fluency.
Fostering a Collaborative Work Culture
- Human-AI Interaction Skills: As AI tools become standard in the workplace, employees will need training not just in technical skills but also in how to work effectively with AI. This includes understanding AI’s limitations, recognizing biases, and learning to validate AI-generated insights.
- Promoting Empathy and Adaptability: In a world of rapidly evolving technology, soft skills like adaptability, emotional intelligence, and problem-solving will be just as important as technical knowledge. Employers should prioritize these qualities to foster resilience and encourage collaboration between humans and AI.
Equitable Access to AI Opportunities
- Reducing Digital Inequality in the Workforce: Efforts must be made to ensure that the benefits of AI are accessible to a diverse workforce, regardless of socioeconomic status or geographic location. This could include offering subsidized training programs in rural or underserved areas and ensuring AI-related job opportunities reach diverse communities.
- Supporting Workers Through Transition: Workers displaced by AI should have access to support systems, including job placement programs, mental health resources, and financial assistance. These measures can ease the transition and prevent AI-driven inequalities in the labor market.
Expansion Ideas:
- Hybrid Role Certification Programs: Establish certifications for “AI hybrid roles,” validating individuals’ capabilities to work alongside AI, especially in roles that require both technical and non-technical expertise.
- AI-Empowered Freelance Networks: Creating AI-enhanced platforms where freelancers can access AI tools may broaden workforce inclusion, enabling individuals worldwide to participate in AI-driven work.
Conclusion:
“The Ethical AI Framework: Guide to Global Ethics and Standards” is designed to inspire responsible AI practices worldwide. By establishing clear ethical principles and practical standards, this framework invites collaboration and accountability among developers, policymakers, and communities. As AI continues to evolve, so too must our commitment to ensuring it serves humanity’s best interests—promoting fairness, sustainability, and inclusivity. Together, we can foster an AI-driven future that aligns with shared human values and positively shapes our world.